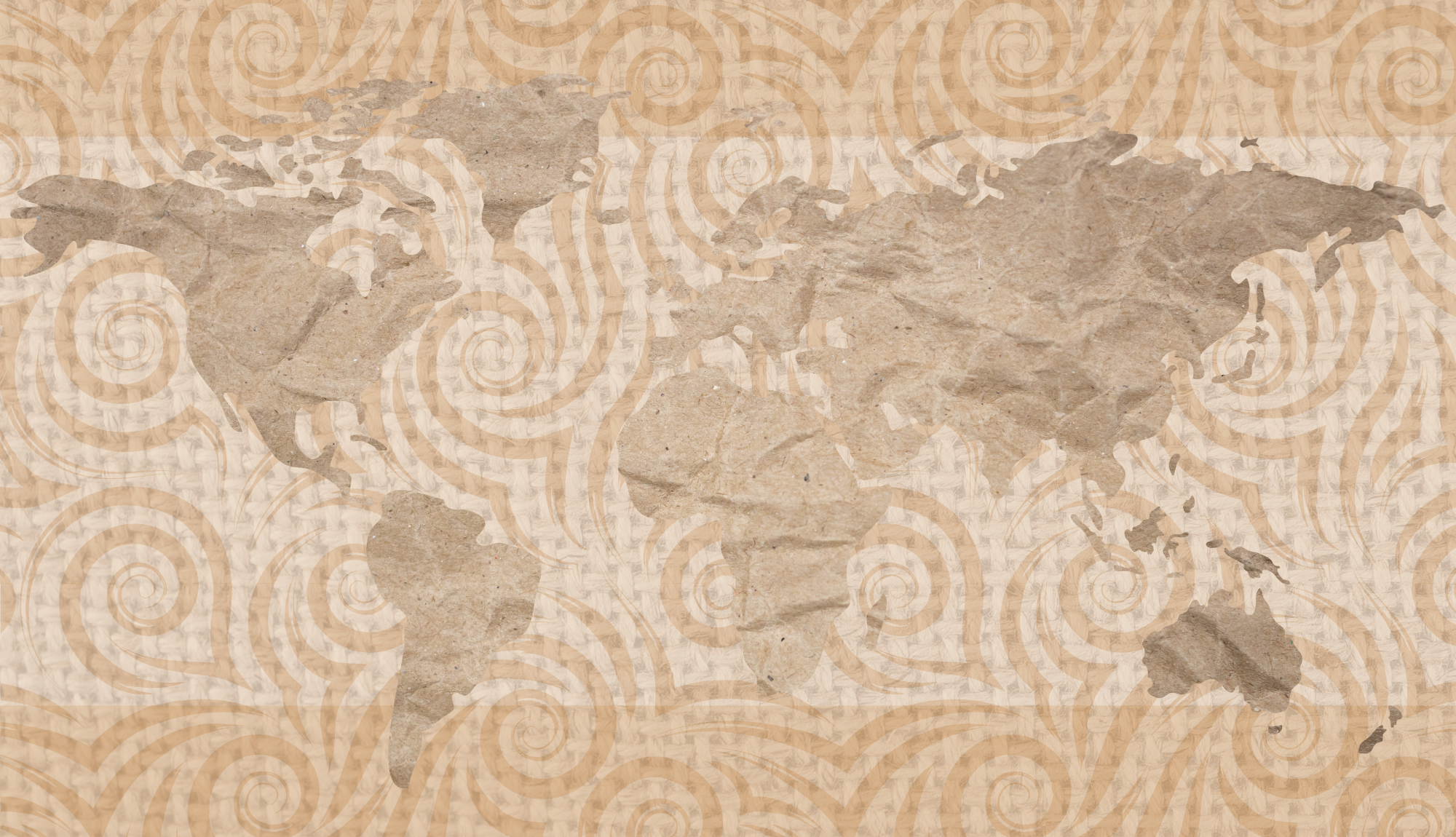
Conference\
2024 Conference on Global Learning
The conference will showcase the full range of global learning activities, programs, strategies, and approaches used by educators worldwide to prepare students for work, life, and citizenship in our interconnected world.